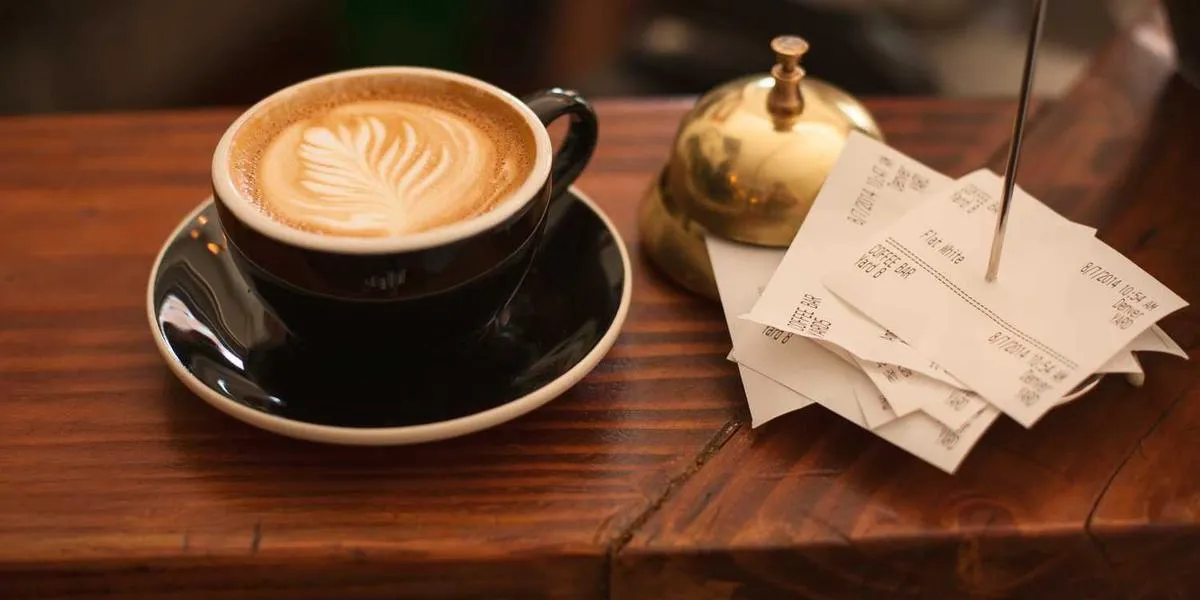
Unsloth's Fine-Tuning Guide: Empowering AI Customization
🤖 AI-Generated ContentClick to learn more about our AI-powered journalism
+Introduction
In the rapidly evolving landscape of artificial intelligence, large language models (LLMs) have emerged as powerful tools capable of tackling a wide range of tasks. However, these models often require fine-tuning to achieve optimal performance and inject domain-specific knowledge. Recognizing this need, Unsloth, a pioneering AI company, has released a comprehensive guide on fine-tuning LLMs, empowering users to tailor these AI systems for their specific requirements.
The Essence of Fine-Tuning
Fine-tuning is a process that involves customizing pre-trained LLMs to enhance their performance and inject new knowledge for specific tasks or domains. This approach has proven to be highly effective, as evidenced by the success of models like ChatGPT-4, which was fine-tuned from GPT-4 to excel at understanding and responding to instructions.
Fine-tuning an LLM customizes its behavior, enhances + injects knowledge, and optimizes performance for domains/specific tasks.
By fine-tuning LLMs, users can tailor these models to excel in specific domains, such as legal analysis, medical research, or creative writing, among others. This process not only enhances the model's performance but also injects domain-specific knowledge, ensuring that the AI system can provide more accurate and relevant outputs.
Unsloth's Accessible Approach
One of the standout features of Unsloth's fine-tuning guide is its emphasis on accessibility. The company recognizes that fine-tuning LLMs often requires significant computational resources, which can be a barrier for many users. To address this challenge, Unsloth offers free tools that enable fine-tuning on platforms like Colab and Kaggle, requiring minimal hardware resources.
With Unsloth, you can fine-tune for free on Colab, Kaggle, or locally with just 3GB VRAM by using our notebooks.
By democratizing access to fine-tuning capabilities, Unsloth aims to empower a broader range of users, from researchers and developers to small businesses and individuals, to harness the full potential of LLMs.
The Fine-Tuning Process
Unsloth's guide provides a comprehensive walkthrough of the fine-tuning process, starting with selecting the right model and fine-tuning method. The company recommends beginning with smaller models and considering efficient methods like LoRA and QLoRA, which can significantly reduce the computational requirements for training.
One of the critical steps in fine-tuning is creating a high-quality dataset that accurately represents the target domain or task. The guide offers insights into data collection, preprocessing, and augmentation techniques to ensure optimal model performance.
Additionally, the guide delves into the intricacies of hyperparameter tuning, a crucial aspect of fine-tuning that can significantly impact model performance. By providing recommendations on batch sizes, learning rates, and other hyperparameters, Unsloth aims to help users strike the right balance between effective learning and avoiding overfitting or underfitting.
Evaluating and Deploying Fine-Tuned Models
Once the fine-tuning process is complete, Unsloth's guide offers insights into evaluating the performance of the fine-tuned model. This includes techniques for measuring model accuracy, assessing generalization capabilities, and identifying potential biases or limitations.
Finally, the guide covers the steps for running the fine-tuned model, saving it, and exporting it for use in various inference engines. This ensures that users can seamlessly integrate their fine-tuned models into their applications or workflows, unlocking new possibilities for AI-powered solutions.
Community Engagement and Support
Recognizing the importance of community support and continuous learning, Unsloth encourages users to explore additional fine-tuning tips and resources on their blog. The company has also established a vibrant community where users can seek guidance, share experiences, and collaborate on fine-tuning projects.
Ai has personality. We're all doomed
As the fine-tuning guide gains traction within the AI community, discussions and debates have emerged, reflecting the excitement and concerns surrounding the rapid advancements in this field. While some users embrace the potential of fine-tuned LLMs with enthusiasm, others express apprehension about the implications of increasingly capable AI systems.
While I don’t agree with his trust in our government protections, he’s right to point out that an immense amount of data has been collected on each of us and is out there. Companies like OpenAI will not stop until they’ve collected every scrap.
As the capabilities of LLMs continue to expand, concerns surrounding privacy, data collection, and the potential misuse of these powerful AI systems have come to the forefront. While Unsloth's guide focuses on the technical aspects of fine-tuning, it is clear that the broader implications of AI advancements will require ongoing dialogue and responsible development practices.
Conclusion
Unsloth's fine-tuning guide represents a significant step forward in empowering users to harness the full potential of LLMs. By providing accessible tools and comprehensive guidance, the company is democratizing the process of fine-tuning, enabling a broader range of users to tailor these powerful AI systems to their specific needs.
As the AI landscape continues to evolve, the ability to fine-tune LLMs will become increasingly crucial for unlocking new applications and driving innovation across various domains. Unsloth's guide serves as a valuable resource for navigating this exciting frontier, empowering users to shape the future of AI and unlock its transformative potential.