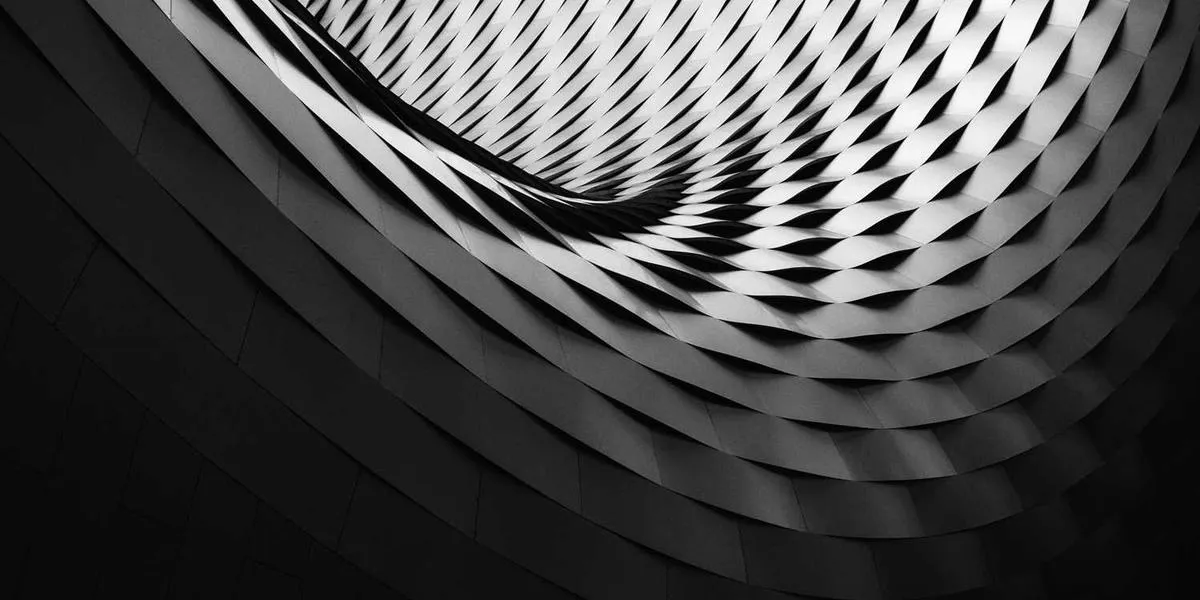
Meta's Web-SSL Challenges Language-Supervised Visual Learning
🤖 AI-Generated ContentClick to learn more about our AI-powered journalism
+Introduction
The field of computer vision and multimodal learning has been dominated by language-supervised models like CLIP, which leverage the power of natural language to guide the learning of visual representations. However, a recent breakthrough from Meta AI has challenged this paradigm, reigniting the debate around the necessity of language supervision for achieving strong visual understanding.
Meta AI has released the Web-SSL family of models, a scalable and language-free approach to visual representation learning. By training vision-only models on web-scale data, Web-SSL demonstrates that it can match and even surpass the performance of language-supervised methods like CLIP across a wide range of visual question answering (VQA) tasks and traditional vision benchmarks.
By scaling model size and training data, we show that vision-only models can match and even surpass language-supervised methods like CLIP.
The Web-SSL Approach
Web-SSL encompasses two visual self-supervised learning (SSL) paradigms: joint-embedding learning (via DINOv2) and masked modeling (via MAE). These models are trained exclusively on the image subset of the MetaCLIP dataset (MC-2B), a web-scale dataset comprising two billion images. This controlled setup enables a direct comparison between Web-SSL and CLIP, both trained on identical data, isolating the effect of language supervision.
To explore the capabilities of language-free visual learning at scale, Meta has released the Web-SSL family of DINO and Vision Transformer (ViT) models, ranging from 300 million to 7 billion parameters, now publicly available via Hugging Face. These models are trained exclusively on the image subset of the MetaCLIP dataset (MC-2B)—a web-scale dataset comprising two billion images. This controlled setup enables a direct comparison between WebSSL and CLIP, both trained on identical data, isolating the effect of language supervision.
Scaling Up Visual SSL
One of the key findings of the Web-SSL research is the scalability of visual SSL models. As the authors scaled up the model size and training data, the performance of Web-SSL continued to improve, surpassing that of CLIP models and showing no signs of saturation even at 7 billion parameters.
Visual SSL models scale better than CLIP models in terms of data and model capacity, and visual SSL performance does not saturate even after scaling up to 7B parameters.
This finding challenges the prevailing belief that language supervision is a crucial component for achieving strong visual representation learning. By demonstrating that vision-only models can match and even exceed the performance of language-supervised models when properly scaled, Web-SSL opens up new avenues for exploring vision-centric representation learning without the need for language supervision.
Will be embarrassing for Meta if this ends up clowning Maverick
Implications and Future Directions
The success of Web-SSL has significant implications for the field of computer vision and pattern recognition. It suggests that the integration of language in visual pretraining, while beneficial, might not be the sole factor driving the superior performance of multimodal models like CLIP. Instead, the capacity to scale visual SSL models effectively plays a critical role in achieving high levels of performance.
This opens up new avenues for exploring vision-centric representation learning without the necessity for language supervision, potentially simplifying the model training process and enhancing the accessibility of high-quality visual representation models. Additionally, the Web-SSL research highlights the importance of data distribution and the potential for further improvements by curating datasets with higher concentrations of text-rich images for tasks like OCR and chart understanding.
Wow, it is just like me at work, maybe it IS ready to replace me.
Community Engagement and Open-Source Contributions
Meta AI's decision to release the Web-SSL models and code through open-source channels like GitHub and Hugging Face has fostered community engagement and collaboration. Researchers and developers can now experiment with these models, integrate them into their applications, and contribute to further advancements in the field.
The Web-SSL project acknowledges contributions and collaborations with teams and individuals across FAIR, Meta, and the broader research community, underscoring the collective effort in advancing visual representation learning. This open-source approach aligns with the principles of transparency and reproducibility in AI research, enabling others to validate and build upon the findings.
Sure do. It's nice knowing the root cause and actually be able to fix it instead of taking more BP meds. I feel like it literally just saved my life.
Conclusion
Meta AI's Web-SSL research has ignited a fascinating debate within the AI community, challenging the long-held belief that language supervision is a prerequisite for achieving strong visual representation learning. By demonstrating the scalability and performance of vision-only models, Web-SSL has opened up new possibilities for exploring vision-centric approaches that could potentially simplify model training and enhance accessibility.
While the integration of language has undoubtedly been beneficial for multimodal models like CLIP, Web-SSL suggests that the capacity to scale visual SSL models effectively is a crucial factor in achieving high levels of performance. As the research community continues to explore and build upon these findings, we can expect further advancements in computer vision and pattern recognition, potentially unlocking new applications and use cases.
Meta AI's commitment to open-source collaboration and transparency has fostered community engagement and enabled researchers and developers to contribute to the ongoing progress in this field. As the Web-SSL models and code become more widely adopted and integrated, we can anticipate exciting developments that push the boundaries of what is possible with vision-centric representation learning.