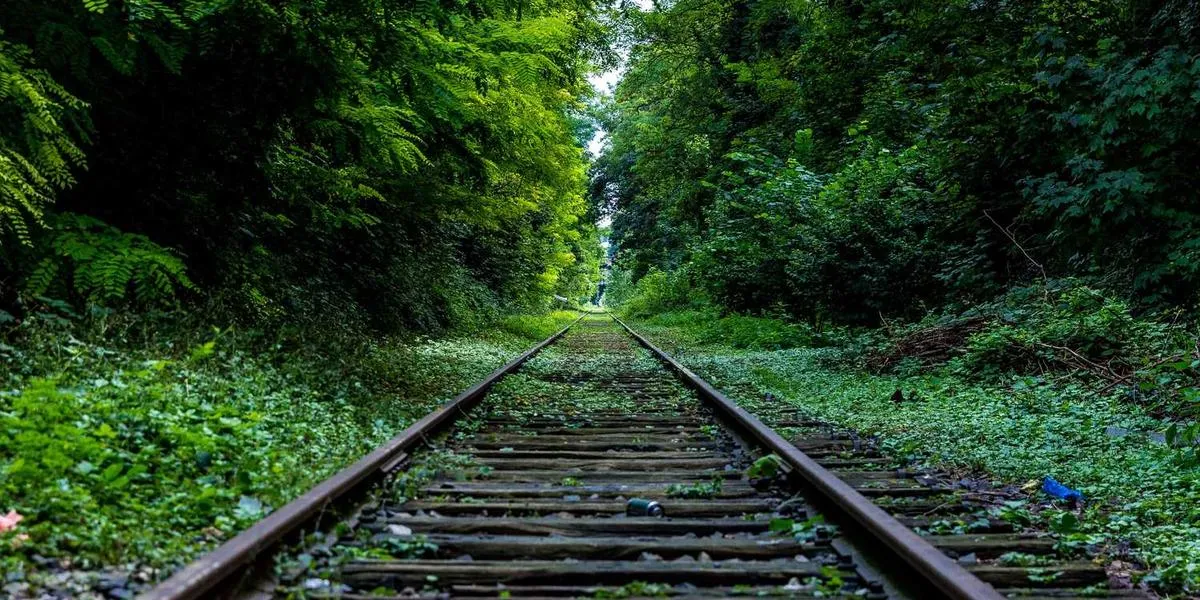
Chain-of-Thought Prompting: Unlocking Reasoning in Large Language Models
🤖 AI-Generated ContentClick to learn more about our AI-powered journalism
+Introduction
In the rapidly evolving landscape of artificial intelligence, large language models (LLMs) have emerged as powerful tools capable of tackling a wide range of tasks. However, their ability to perform complex reasoning has been a longstanding challenge. Recent research conducted by Jason Wei and colleagues at the University of Washington and the Allen Institute for AI has unveiled a simple yet remarkably effective technique to enhance the reasoning capabilities of these models: chain-of-thought prompting.
We explore how generating a chain of thought -- a series of intermediate reasoning steps -- significantly improves the ability of large language models to perform complex reasoning.
By providing LLMs with a few exemplars of step-by-step reasoning as prompts, the researchers demonstrated that these models can naturally improve their performance across a variety of tasks, including arithmetic, commonsense, and symbolic reasoning. The study highlights empirical evidence of the benefits of this simple method, showcasing remarkable improvements in accuracy, particularly noted on the GSM8K benchmark for math word problems, where a 540B-parameter model outperformed the fine-tuned GPT-3 model with a verifier, setting a new state-of-the-art record.
The Power of Chain-of-Thought Prompting
The concept of chain-of-thought prompting is deceptively simple: by providing LLMs with a few examples of step-by-step reasoning as prompts, the models can learn to generate their own chains of thought, breaking down complex problems into smaller, more manageable steps. This approach leverages the models' ability to learn from examples and adapt to new tasks, enabling them to reason through problems in a more structured and transparent manner.
Chain-of-thought prompting significantly enhances the reasoning abilities of large language models across various tasks.
The researchers evaluated the effectiveness of chain-of-thought prompting across a diverse set of tasks, including arithmetic word problems, commonsense reasoning, and symbolic operations. The results were remarkable, with the prompted models consistently outperforming their non-prompted counterparts, often by substantial margins.
Pushing the Boundaries of Math Word Problems
One of the most notable achievements of the study was the performance of the prompted 540B-parameter model on the GSM8K benchmark for math word problems. This benchmark has long been a challenging task for language models, requiring a combination of natural language understanding, quantitative reasoning, and step-by-step problem-solving.
A 540B-parameter language model, prompted with just eight chain of thought exemplars, achieved state-of-the-art accuracy on the GSM8K math word problem benchmark.
Remarkably, the prompted model surpassed the performance of even fine-tuned GPT-3 models with a verifier, setting a new state-of-the-art record on this challenging benchmark. This achievement underscores the potential of chain-of-thought prompting to unlock the reasoning capabilities of LLMs without the need for extensive fine-tuning or task-specific training.
The method surpasses the performance of even finetuned GPT-3 models with a verifier, indicating a notable empirical gain.
Implications and Future Directions
The success of chain-of-thought prompting has far-reaching implications for the development and deployment of LLMs. By enhancing the reasoning capabilities of these models, a wide range of applications, from question-answering systems to decision support tools, could benefit from more transparent and explainable decision-making processes.
I feel like this is their goal with the latest update. Adding a long term memory makes sense if you want your ai to be a long term companion to the user.
Furthermore, the simplicity of chain-of-thought prompting makes it a highly accessible technique, potentially enabling a broader range of researchers and developers to leverage the reasoning capabilities of LLMs without the need for extensive computational resources or specialized expertise.
As the field of AI continues to evolve, it is likely that chain-of-thought prompting will serve as a foundation for further research and development, potentially leading to more advanced techniques for enhancing the reasoning abilities of LLMs. Additionally, the study's findings may inspire new approaches to model training and prompt engineering, further expanding the capabilities of these powerful models.
Conclusion
The research conducted by Jason Wei and colleagues has unveiled a simple yet powerful technique that has the potential to reshape the way we approach reasoning tasks with large language models. By leveraging chain-of-thought prompting, these models can now tackle complex problems with greater transparency and accuracy, paving the way for a wide range of applications that demand robust reasoning capabilities. As the field of AI continues to evolve, it will be fascinating to witness the further development and adoption of this technique, as well as the emergence of new approaches that build upon its foundations.